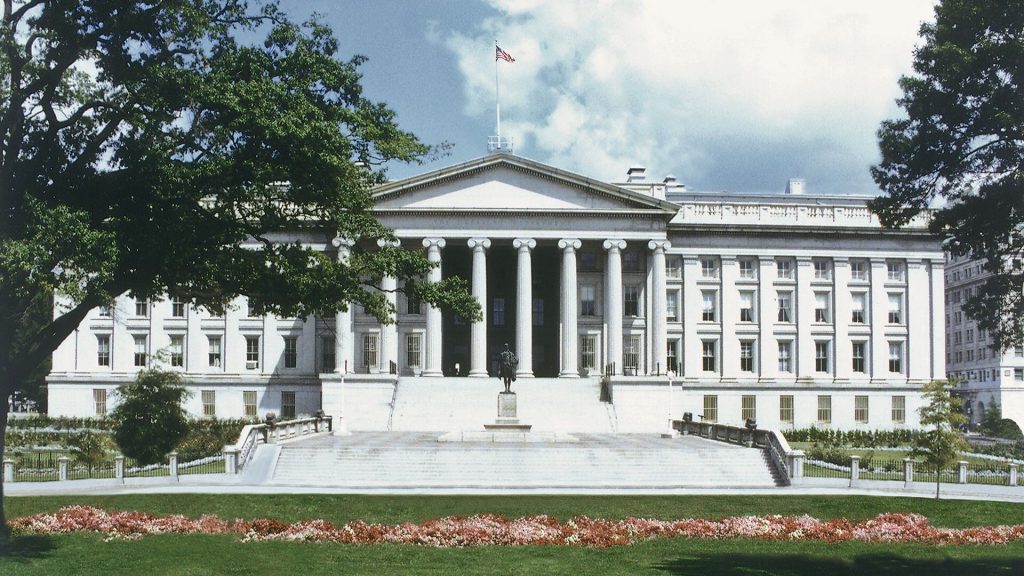
Artificial Intelligence (AI) is transforming industries worldwide, and the financial services sector is no exception. However, with rapid advancements come emerging risks that require immediate attention. This post, based on the U.S. Department of the Treasury's report, addresses the critical AI-related cybersecurity and fraud risks in financial services and offers best practices for navigating this evolving landscape.
The Growing Role of AI in Financial Services
AI is already deeply embedded in the operations of many financial institutions, particularly for cybersecurity and fraud detection. Early adopters continue to explore new use cases as technology advances, with Generative AI standing out as a particularly powerful but risky innovation. However, the adoption of AI in financial services presents unique risks, requiring robust risk management frameworks.
Top AI Cybersecurity Risks
- Data Integrity and Poisoning: AI models rely heavily on data for training and testing. This dependency makes them vulnerable to data poisoning, where malicious actors inject corrupted data to influence model behavior. Financial institutions must safeguard the data pipeline at every stage of the AI lifecycle.
- Data Leakage: During model inference, sensitive information may inadvertently be exposed. Robust encryption, data masking, and rigorous data management protocols are essential for reducing these risks.
- Model Manipulation: AI models can be exploited through adversarial attacks, where attackers manipulate inputs to deceive the AI into making incorrect predictions, a particular risk for fraud detection models.
Fraud Risks and the Need for Data Collaboration
Fraud detection is another critical area where AI has shown great potential, but this success hinges on the availability of large, high-quality datasets. One significant issue identified in the report is the lack of fraud data sharing among financial institutions, especially for smaller organizations. Larger institutions tend to have more comprehensive data to train fraud detection models, creating a gap between large and small financial entities.
To address this, initiatives by organizations like the American Bankers Association (ABA) and Treasury’s Financial Crimes Enforcement Network (FinCEN) are pushing for better data-sharing frameworks to improve AI-driven fraud detection for all financial institutions.
Regulatory Considerations
The current regulatory landscape for AI in financial services is evolving, with key regulatory bodies like the Financial Stability Oversight Council (FSOC) and the National Institute of Standards and Technology (NIST) leading the way. The U.S. Department of the Treasury report emphasizes the importance of integrating AI risk management into broader enterprise risk management frameworks. This ensures that financial institutions can maintain compliance while using AI systems securely.
Best Practices for Managing AI-Specific Cybersecurity Risks
- Embed AI Risk Management into Enterprise Programs: Financial institutions should align AI risk management with broader enterprise risk frameworks to ensure that AI-related risks are governed comprehensively.
- Develop Tailored AI Risk Frameworks: Many institutions are developing bespoke AI risk frameworks, leveraging existing guidelines like the NIST AI Risk Management Framework (RMF), to identify and mitigate AI-specific risks.
- Enhance Data Governance: With AI's reliance on data, financial institutions must strengthen data governance, ensuring that all data used for training and inference is clean, secure, and free from bias.
The Role of vCISO/Field CISO in AI Risk Management
In parallel, the virtual CISO (vCISO) or Field CISO offers strategic oversight for integrating AI into an organization’s cybersecurity framework. These professionals ensure that AI-related risks are managed holistically across the organization, providing the necessary guidance to align AI initiatives with regulatory requirements and industry best practices.
Key roles of a vCISO in managing AI-specific risks include:
- AI Risk Assessment and Strategy: A vCISO helps organizations assess AI-related risks and develop a cybersecurity strategy that incorporates AI tools while mitigating potential vulnerabilities. This includes advising on the ethical use of AI, data governance, and privacy concerns, especially in regulated industries like financial services.
- Regulatory Compliance: As AI is increasingly subject to regulatory scrutiny, vCISOs ensure that AI systems comply with relevant laws, including those related to data protection, cybersecurity, and anti-fraud measures. Their expertise is vital in helping financial institutions navigate complex regulatory landscapes while leveraging AI’s full potential.
- Cross-Enterprise Collaboration: AI cybersecurity requires collaboration across multiple teams, including IT, legal, and compliance. A vCISO facilitates this collaboration, ensuring that AI risk management is integrated into the broader enterprise risk management framework.
- Third-Party Risk Management: Many financial institutions rely on third-party AI solutions for fraud detection, cybersecurity, or both. The vCISO plays a critical role in evaluating these vendors, ensuring that they meet the institution’s security requirements and do not introduce additional risks.
Looking Ahead
The financial services sector’s adoption of AI is set to continue growing, but so too are the associated risks. By implementing robust AI-specific risk management frameworks and fostering collaboration across the sector, financial institutions can mitigate the risks and harness the full potential of AI to enhance cybersecurity and fraud detection capabilities.
Contact Critical Path Security to learn more about how we can help secure your AI-powered future.